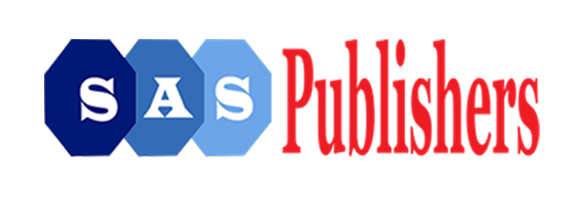
An International Publisher for Academic and Scientific Journals
Author Login
Scholars Journal of Economics, Business and Management | Volume-5 | Issue-01
Trend and Regression Analysis of Stock Market Price of Select Industries in India
V. Richard Paul, K. Somanathan
Published: Jan. 30, 2018 |
166
161
DOI: 10.36347/sjebm.2018.v05i01.003
Pages: 17-23
Downloads
Abstract
The base structure for every large scale industry is finance. The stock market remains a source of investment to raise financial status through an issue of share. Investors receive return from their investment. Stock prices are often very tempting in an economic downturn. Share price is the index of company’s financial performance. The present study analyses the trend and regression of stock market price of select industries in India. There are number of reviews previously available related to stock market price analysis of companies. But there is limited study available on industry-wise stock market price analysis. Hence the paper has attempted the trend and regression analysis of stock price of select industries in Bombay Stock Exchange in India. The study is based on only secondary data and data collected for a period of 10 years from 2007-2016. Among the eighty six different industries the researcher has selected fifteen industries on the basis of convenient sampling method. Four companies in each industry which have market capitalization of Rs.200 crores and above have been selected for the analysis. The entire data is evaluated by using SPSS and Microsoft Excel software. This paper also finds forecast trend in the select Industries share price of growth by analyzing polynomial trend based on the forecasted trend pattern. The cubic trend method is applied in the first part of the paper. Among the 15 industries nine have positive growth and significant F value at 5% level except construction, fertilizer, hotel, steel and textiles industries. The second part of this paper estimates regression analysis of share price of select industries. It is to ascertain how collinearity in general, and the sign of correlations in particular, affect parameter inference, variable omission bias and their diagnostic indices in regression. The test is classified by included and excluded variables based on collinearity correlation relationship. The findings indicate a strong re