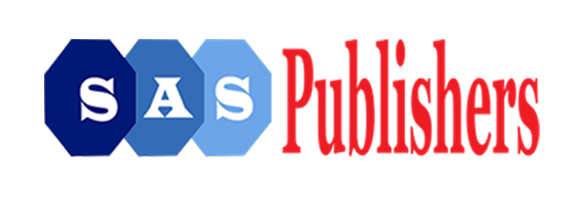
An International Publisher for Academic and Scientific Journals
Author Login
Scholars Journal of Engineering and Technology | Volume-12 | Issue-07 Call for paper
A Long Short-Term Memory Network for Product Quality Monitoring in Fused Deposition Modeling
Md Emon Ahmed, Nur Mohammad Ali, Mohammad Shah Paran, Abdur Rahman, Jayanta Bhusan Deb
Published: July 24, 2024 |
25
28
DOI: 10.36347/sjet.2024.v12i07.004
Pages: 230-241
Downloads
Abstract
This study investigated the influence of room temperature on the final prototype parts produced using the fused deposition modeling (FDM) technique. Real-time room temperature data was collected using a wireless sensor with a time series data collection method. A data-driven model called Long Short-Term Memory (LSTM) network was developed to predict room temperature. The model was trained using data from the wireless sensors collected during the experimental procedure, which involved printing prototype parts in different seasons of the year. The developed LSTM network demonstrated its capability to accurately predict temperature, enabling the detection of printing defects under various room temperature conditions. The study revealed that lower room temperatures had a more significant impact on the surface roughness of the printed parts compared to higher room temperatures. The effectiveness of the developed model was confirmed by comparing its results with the experimental data using Root Mean Square Error (RMSE). The developed LSTM model found an RMSE of 0.003993 for predicting cold room temperature data and an RMSE of 0.033993 for predicting hot temperature data. The developed LSTM model offers a valuable tool for detecting printing defects in different room temperature conditions. It provides users with information about the room temperature necessary for printing high-quality parts, thereby enhancing the printing process capability and minimizing defect issues in the printed parts.