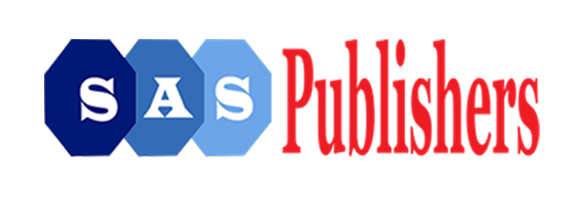
An International Publisher for Academic and Scientific Journals
Author Login
Scholars Journal of Engineering and Technology | Volume-12 | Issue-12
Application of AI Algorithms for the Prediction of the Likelihood of Sickle Cell Crises
Essang Samuel Okon, Kolawole Olamide Michael, Runyi Emmanuel Francis, Ante Jackson Efiong, Ogar-Abang Micheal Obi, Auta Jonathan Timothy, Okon Paul Edet, Effiong Raphael Dominic, Ukim Akanimo Jimmy
Published: Dec. 26, 2024 |
79
76
DOI: https://doi.org/10.36347/sjet.2024.v12i12.008
Pages: 394-403
Downloads
Abstract
This paper investigates the application of Support Vector Machines (SVM), Random forest and Logistic Regression as AI algorithms to predict the likelihood of sickle cell crises. The study focused on the various considerations involved in utilizing SVMs, Random forest and Logistic Regression for this predictive task, encompassing feature selection, data quality assessment, handling class imbalance, model training, hyper parameter optimization, evaluation metrics, and interpretability. Special attention is given to addressing the complexities of Sickle Cell Disease data and ensuring the reliability of predictions. Through a comprehensive analysis, we accentuate the relevance of SVMs, Random forest and Logistic Regression in capturing intricate relationships within high-dimensional SCD datasets. It emphasizes the importance of feature selection, particularly in integrating genetic markers, clinical parameters, and environmental factors, to enhance prediction accuracy. Also, we highlight the significance of model interpretability in healthcare applications, enabling clinicians to understand the rationale behind predictions and facilitating informed decision-making. Validation and testing procedures are highlighted as crucial steps to ensure the generalizability and robustness of the SVM, Random Forest and Logistic Regression-based predictive model in real-world clinical settings. Finally, leveraging Support Vector Machines and other machine learning algorithms for predicting sickle cell crises likelihood offers promising avenues for proactive management and personalized care strategies. By harnessing AI algorithms effectively, healthcare practitioners can enhance patient outcomes through timely interventions tailored to individual risk profiles. However, further research and validation efforts are warranted to maximize the clinical utility and reliability of SVM, Random forest and Logistic Regression -based predictive models in SCD management.