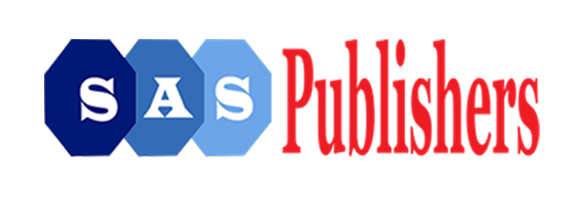
An International Publisher for Academic and Scientific Journals
Author Login
Scholars Journal of Physics, Mathematics and Statistics | Volume-7 | Issue-02
On Using Multivariate Nonparametric Regression Methods
Ahmed M. Mami, Maryam M. Mansour, Abobaker M. Jaber
Published: Feb. 23, 2020 |
140
203
DOI: 10.36347/sjpms.2020.v07i02.001
Pages: 11-29
Downloads
Abstract
Nonparametric regression analysis refers to the absent of the regression estimation of the parameters. There are many drawbacks of using the parametric modeling due to the restrictions imposed on the regression function, as well as the lack of flexibility provided. The answer to the rigidity of parametric regression is to assume that the regression function f does not belong to a parametric family. This will lead us to the nonparametric regression modelling. The scope of nonparametric regression is very broad, ranging from "smoothing" the relationship between two variables in a scatter plot to multivariate regression analysis where we encounter what so called" the curse of dimensionality". This paper aims to propose two methods of the nonparametric regression, namely; the Multivariate Local Polynomial Kernel (MLPK) Regression, and Multivariate Regression Splines (MRS). The theoretical development of these two nonparametric methods, that includes the asymptotic bias, variance, and mean squared errors are presented. Then, data-driven methods of bandwidth selection of the two multivariate nonparametric estimators are derived. Several simulation studies are conducted in order to evaluate and compare the estimated regression curves resulting from utilizing two different classes of the Multivariate Nonparametric regression curve estimation procedures, namely: MLPK estimator, and MRS estimator. Such a study is necessarily to be restrictive, because there are many possibilities for the number of explanatory variables d, the choices of regression functions f(x), sample size (n), and the choice of σ. In this study, we conducted three main simulation studies depending upon d=1, d=2, and d=3. Several interesting results have been achieved. Applications on real dataset also been considered. The dataset used is the Cross Country Growth Panel Data. Lastly, the advantages and disadvantages of these two nonparametric regression estimators have been discussed.