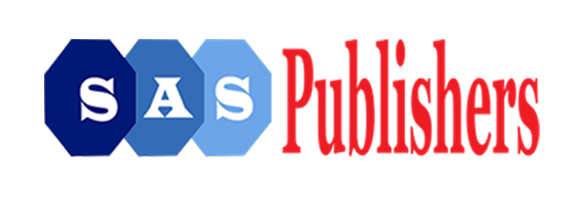
An International Publisher for Academic and Scientific Journals
Author Login
Scholars Journal of Physics, Mathematics and Statistics | Volume-8 | Issue-09
Parameters Estimation Sensitivity of the Linear Mixed Model To Alternative Prior Distribution Specifications
Nasir Elmesmari, Farag Hamad, Abdelbaset Abdalla
Published: Nov. 11, 2021 |
121
89
DOI: 10.36347/sjpms.2021.v08i09.001
Pages: 166-170
Downloads
Abstract
Markov chain Monte Carlo (MCMC) is the most widely used method for estimating joint posterior distributions in Bayesian analysis. The Markov chain Monte Carlo technique has been used in order to estimate the model parameters based on the different prior distributions. MCMC simulations were carried out in order to evaluate the linear mixed model using different parameters of the prior distribution. In this paper, we established the linear mixed model with different types of variables. The proposed parameters of the prior distribution are different from the traditional parameters of the prior distribution. We assumed special parameters for the prior distribution based on some background or information about the data science. This work aims to estimate the parameters using a point estimator or find a confidence interval (credible interval) for the unknown parameters. Also, a specific hypothesis about these parameters can be tested using a random sample from the posterior distribution. The performance of each prior is measured based on the effective sample size (ESS) for the estimated model. The results showed that the estimated linear mixed model with proposed parameters of the prior distribution performed very well in comparison with the standard or traditional prior (inverse-Wishart prior for random effect component). Based on the scale reduction factors, the estimated model with proposed parameters performed better in comparison with scale reduction factors for the traditional model.