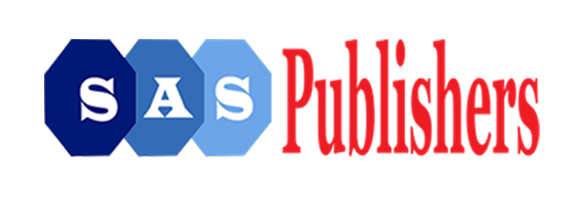
An International Publisher for Academic and Scientific Journals
Author Login
Scholars Journal of Physics, Mathematics and Statistics | Volume-9 | Issue-04
Quantile Regression-based Multiple Imputation of Skewed Data with Different Percentages of Missingness
Nwakuya, M. T, Onyegbuchulam B. O.
Published: May 10, 2022 |
133
74
DOI: 10.36347/sjpms.2022.v09i04.002
Pages: 41-45
Downloads
Abstract
This study investigates the Quantile Regression-Based Multiple Imputation (QR-based MI) on a simulated right skewed data with 5% and 25% missing data points. Quantile regression analysis on three data sets that comprises of the complete skewed data without missing values, data set with 5% missing values and data set with 25% missing values was performed at 0.25, 0.5, 0.75 and 0.95 quantiles. The data sets with 5% and 25% missing values were imputed using QR-based MI technique, giving rise to two complete data sets. This analysis was performed using both transformed and untransformed version of the three data sets. The transformation was carried out by applying the Yeo-Johnson transformation technique and comparison of results was based on the Mean Square Error (MSE), Akiake Information Criteria (AIC) and Bayesian Information Criteria (BIC). The result from the original complete right skewed data shows that the untransformed data presented better results at 0.25 and 0.50 quantiles compared to the transformed data while results at 0.75 and 0.95 quantiles of the transformed data showed a better result compared to the untransformed. This result is attributed to the fact that the data was right skewed, so that the transformation will benefit the heavy tails on the right while the lighter tail on the left needs not to be transformed hence the 0.25 and 0.50 quantile better result with untransformed data and the 0.75 and 0.95 better result with transformed data. Considering the imputed complete data sets from the 5% and 25% missingness, it was seen that for both data sets at all quantiles considered, the untransformed data produced better results than the transformed data. This led us to conclude that the QR-based MI is not distribution dependent hence it is not sensitive to skewness. Therefore it can be stated based on the results that QR-based MI is robust to skewness, thus can be applied to skewed data sets.