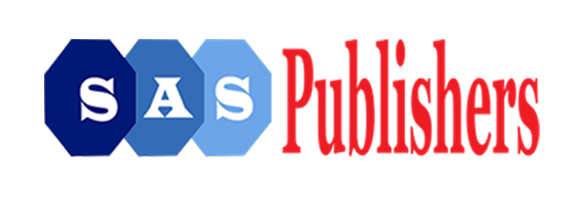
An International Publisher for Academic and Scientific Journals
Author Login
Scholars Journal of Physics, Mathematics and Statistics | Volume-9 | Issue-05
Investigating Performance of Composite Quantile Regression with and without Penalization
Nwakuya, M. T, Nduka, E. C
Published: June 22, 2022 |
122
81
DOI: 10.36347/sjpms.2022.v09i05.002
Pages: 85-91
Downloads
Abstract
The performance of composite quantile regression with and without penalization was investigated and results compared to Lasso Quantile Regression (LQR), Lasso Regression (LR) and Ridge Regression (RR). The mean square error, Akiake information criteria and mean absolute percentage error were used as the comparative criteria. The comparison was illustrated using real dataset and simulated data sets of sample sizes n=30,100,300,500 and 1000. Five consecutive quantiles; 0.19, 0.39, 0.59, 0.79 and 0.99 were used for the quantile regression methods while the lasso regression and ridge regression were based on the mean effect. Another set of quantiles; 0.25, 0.5, 0.75 and 0.95 were also accessed based on only CQR and CQR_AL. The results shows that the composite quantile regression without penalization (CQR) and composite quantile regression with penalization (CQR_AL) achieved same results with the lowest variance on estimated effects and was best fitted model for very large data sets (n=100, 300, 500 & 1000). For the real life data with sample size of 318, the CQR_AL showed the least MSE=421.7653 and AIC=1930.136, but its prediction accuracy was low at 19.03%. Considering sample size 30, the LQR at the 0.59th quantile had the lowest variance with MSE of 1.3940 and was best fitted with AIC of 340.20, also it was noticed that COR_AL achieved the next lowest value for MSE and AIC. These results led to the conclusion that CQR_AL and CQR can be used alternatively with large data sets. But when the sample size is small the LQR is most suited, but if a combined quantile effect is sort the CQR_AL should be opted for.