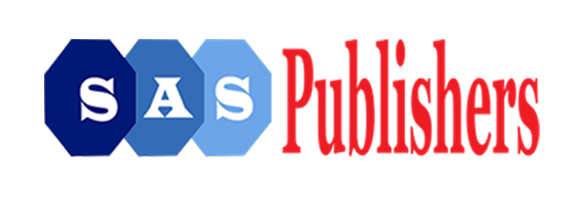
An International Publisher for Academic and Scientific Journals
Author Login
Scholars Journal of Physics, Mathematics and Statistics | Volume-3 | Issue-04
Stochastic Gradient Descent with SVM for Imbalanced Data Classification
Lu Shuxia, Zhu Chenxu, Zhou Mi
Published: Nov. 20, 2016 |
220
189
DOI: 10.36347/sjpms
Pages: 161-165
Downloads
Abstract
Stochastic Gradient Descent (SGD) is an attractive choice for SVM training. SGD leads to a result that the
probability of choosing majority class is far greater than that of minority class for imbalanced classification problem. In
order to deal with the large-scale imbalanced data classification problems, a method named stochastic gradient descent
algorithm with SVM for imbalanced data classification is proposed. First, to deal with imbalanced data classification
problems, we define the weight according to the size of positive and negative dataset. Then, a fast learning algorithm on
large datasets called the weighted stochastic gradient descent algorithm with SVM is proposed, which helps to reduce the
hyperplane offset to the minority class, thus solve the large-scale imbalanced data classification problems. Experimental
results on real datasets show that the proposed method is effective